Unlocking the Power of Labelling Tool Machine Learning for Businesses
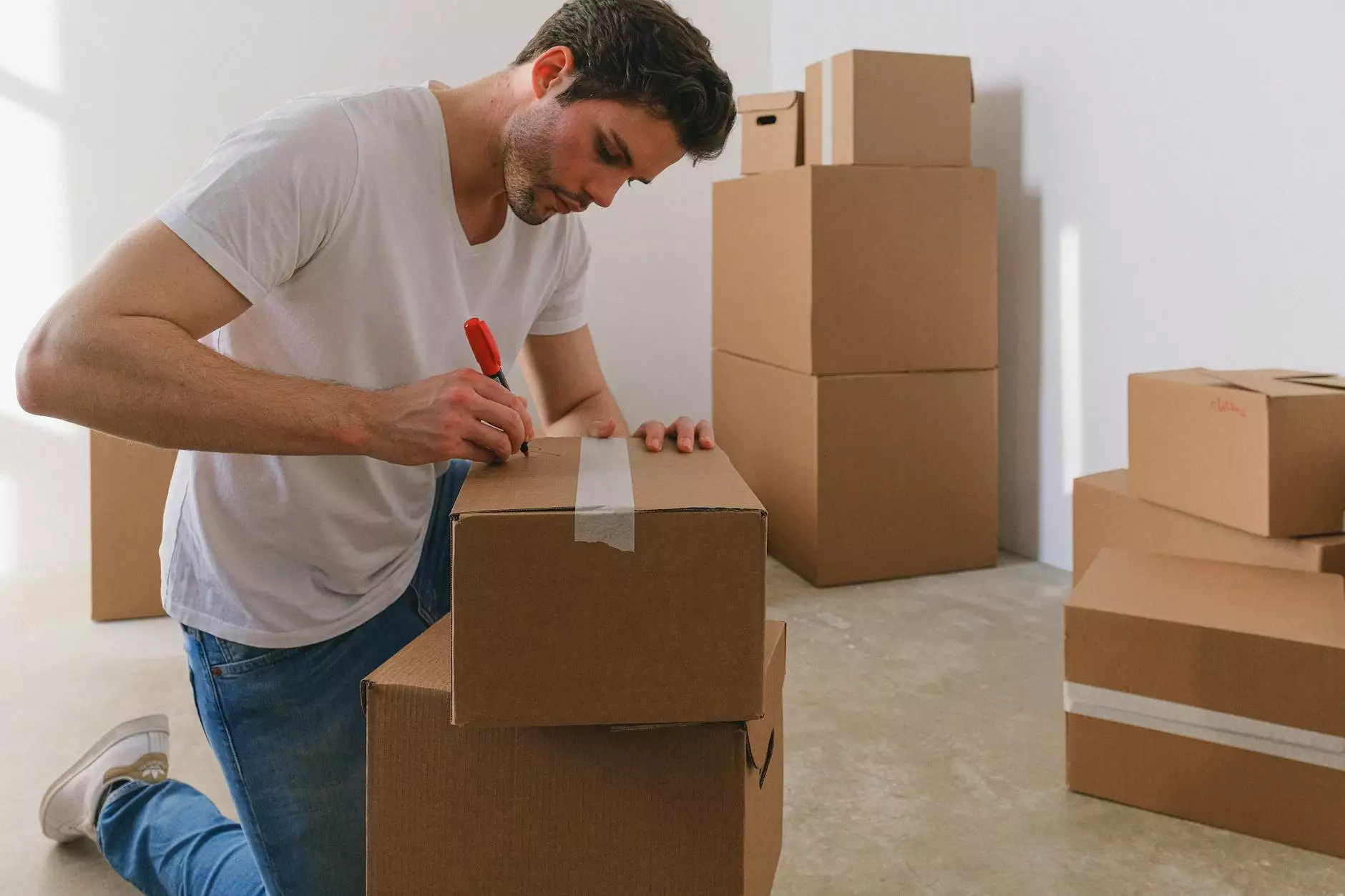
In today's digital age, the sheer volume of data generated daily is staggering. As businesses strive to make sense of this data, the role of labelling tool machine learning becomes increasingly crucial. This technology not only optimizes data annotation processes but also enhances the quality of insights that can be gleaned from raw data. In this article, we will delve into the significance of labelling tools powered by machine learning and how they can significantly benefit businesses across various sectors.
Understanding the Basics: What is a Labelling Tool in Machine Learning?
At its core, a labelling tool machine learning facilitates the transformation of unannotated data into a structured format that machines can understand. This is essential in training machine learning models, which depend on labelled data to learn and make predictions.
- Data Annotation: The process of identifying and tagging data elements within a dataset to provide context and meaning.
- Machine Learning Models: Algorithms that learn patterns and make decisions based on input data.
Labelled data is the foundation upon which machine learning models are built. Without accurate labels, the insights generated by such models may be unreliable, leading to poor business decisions and outcomes.
Why is Data Annotation Important for Businesses?
Data annotation is not just a technical necessity; it is a strategic advantage. Here are some reasons why businesses should invest in robust labelling tools:
- Quality Insights: Well-annotated data leads to more accurate insights and predictions, enabling businesses to make informed decisions.
- Improved Customer Experience: By understanding customer behavior through labeled data, businesses can personalize offerings and enhance satisfaction.
- Competitive Advantage: Companies that leverage machine learning effectively can stay ahead of their competitors by quickly adapting to market changes.
The Role of Labelling Tool Machine Learning in Data Annotation
Labelling tools that integrate machine learning features profoundly change the landscape of data annotation. They work by employing algorithms that can automate parts of the labelling process, significantly reducing the time and effort needed for manual annotations.
Key Features of Advanced Labelling Tools
Advanced labelling tool machine learning solutions offer several features that streamline data annotation:
- Automated Labelling: Machine learning algorithms can learn from existing labelled datasets and apply this knowledge to new data, reducing manual effort.
- Quality Assurance: These tools often include mechanisms to check label accuracy, flagging inconsistencies for human review.
- Scalability: Businesses can handle larger datasets with ease, as machine learning tools can quickly process vast amounts of data.
- Customizability: Many labelling tools allow businesses to customize the labelling schema according to their specific requirements.
Implementing Labelling Tools in Your Business Strategy
Adopting a labelling tool machine learning strategy involves several key steps:
1. Assess Your Data Needs
Identify the types of data your business generates and determine how much of it needs annotation. Different sectors may require different types of labelling, such as image recognition, text categorization, or audio transcription.
2. Choose the Right Labelling Tool
Select a labelling tool that fits your business's specific needs. Factors to consider include:
- Ease of Use: User-friendly interfaces can reduce the learning curve for your team.
- Integrations: The tool should seamlessly integrate with your existing data infrastructure.
- Support and Training: Look for tools that offer comprehensive support and educational resources.
3. Train Your Team
Once the tool is selected, ensure your team is adequately trained. Familiarity with the tool's functionality will enhance efficiency and accuracy in data annotation.
4. Initiate Data Annotation Projects
Start with pilot projects to understand the workflow and make necessary adjustments before scaling up your operations.
5. Continuously Monitor and Improve
Regularly review the effectiveness of your labelling strategies, focusing on data quality and model performance. Adjust your processes and tool usage accordingly.
Case Studies: Success Stories with Labelling Tools
Numerous organizations have successfully implemented labelling tools to enhance their data annotation efforts. Here are a few examples:
Case Study 1: E-commerce Personalization
An e-commerce platform utilized a labelling tool machine learning to analyze customer purchase patterns. By annotating transaction data, the platform could precisely tailor recommendations, resulting in a 30% uplift in sales.
Case Study 2: Healthcare Diagnostics
A healthcare provider used labelling tools to annotate medical imaging data. By training their machine learning models on accurately labelled images, they improved diagnostic accuracy, reducing misdiagnosis rates by 25%.
Case Study 3: Autonomous Vehicles
A leading automotive company employed advanced labelling tools for image and sensor data to train their autonomous driving systems. The meticulous labelling improved their model's ability to recognize objects and make split-second driving decisions.
Challenges in Machine Learning Data Annotation and How to Overcome Them
While the benefits of using labelling tool machine learning are significant, businesses may also face challenges:
1. Data Quality Issues
If the initial unlabelled data is noisy or inconsistent, it will lead to poor results. Ensure you clean and preprocess your data before annotation.
2. Securing Accurate Labels
Inaccurate labelling can lead to misinformed machine learning models. Incorporate quality assurance procedures and utilize feedback loops for continuous improvement.
3. Resource Allocation
Annotation can be resource-intensive. Consider using a combination of automated labelling and human oversight to optimize resource allocation.
The Future of Labelling Tool Machine Learning
The future of machine learning and data annotation is incredibly promising. As technology evolves, we can expect:
- Increased Automation: Greater reliance on AI to automate complex labelling tasks, freeing human resources for higher-level decision-making.
- Enhanced Collaboration: Tools will become increasingly collaborative, allowing teams to work across departments and geographies.
- Integration with Big Data Technologies: Improved integration between labelling tools and big data technologies will streamline the workflow from data generation to insights.
Conclusion
The integration of labelling tool machine learning into business strategies is no longer optional; it is essential for those looking to harness the true power of their data. By investing in advanced annotation tools, businesses can gain quality insights that drive growth, improve customer experiences, and maintain a competitive edge.
Moving forward, companies that prioritize data annotation and invest in the right labelling tools will undoubtedly find themselves at the forefront of innovation and success in an increasingly data-driven world. Ensure that your organization continues to explore and adopt these technologies to stay ahead in the game.